People / Students / Class of 2025
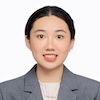
Moyi Li is a recent magna cum laude graduate, having completed her degree in 2024. She holds a Bachelor of Science degree in Applied Mathematics: Data Science, with double minors in Data Science and Informatics, from the University of Washington, Seattle. Throughout her internship and project experiences, her persistent passion for data science has driven her to study and improve continually.
As a Financial Data and Model Analyst Intern, she generated precise payment schedules, compared cash flow through SQL-based operations, and meticulously conducted data mining and analysis to explain the anomalous inconsistencies in three-month delinquency rates across different property states. During her internship, her meticulous work significantly contributed to gaining clearer insights into the influential factors and enhanced the overall accuracy and reliability of financial models. In a personal project, she combines Python, SQL, and Azure to introduce a medical automated appointment reservation system featuring auto-incremented IDs, real-time vaccine inventory updates, and user-friendly appointment verification. She also created a secure account registration system using Azure’s cloud database for real-time password management, employing robust salting, hashing techniques, and stringent password verification measures with a synchronized real-time schedule-search system. In a group project on EdX student model training and evaluation, she employed multiple machine learning models, including Logistic Regression, K-Nearest Neighbors, Decision Trees, Random Forests, AdaBoost, and Neural Networks, to predict course completion using anonymized student data. Throughout the project, she preprocessed a dataset of 10,000 records, performed a train-test split, and enhanced model performance through hyperparameter fine-tuning using Grid Search and ElasticNet techniques. Additionally, she created visualizations such as heatmaps, confusion matrices, and line plots, and conducted a comparative analysis of train accuracy versus validation accuracy across different models. Ultimately, her work achieved a 98.2% prediction accuracy in a Kaggle competition, securing first place among 81 teams.
As a new member of the MLDS program, she aims to bring her professional experiences and unique perspective to the program. She looks forward to engaging with fellow data scientists, sharing insights on advanced analytics and algorithm optimization, and mutually contributing to the community.