Panel Explores How to Maximize AI’s Scientific Benefits and Social Value
Presented by AI@NU, the event featured leaders in AI from Northwestern, academia, and industry

In August 2019, a group of nearly 100 leading artificial intelligence (AI) researchers from academia and industry released a roadmap for AI research and development for the next two decades. The researchers unveiled three recommendations: create and operate a national AI infrastructure to serve academia, industry, and government; re-conceptualize and train an all-encompassing AI workforce to build upon the national AI infrastructure; and stress that core programs for basic AI research are critical.
On October 13, Northwestern University’s AI@NU community held a virtual panel discussion to evaluate the progress and prospects for each of the three major recommendations. The AI@NU Community is led by Kristian Hammond, Bill and Kathy Osborn Professor of Computer Science, and a group of AI researchers and users across campus with the goal of connecting and enhancing AI research at Northwestern.
The panel, focused on core AI programs and how to maximize AI’s scientific benefit and societal value, was moderated by Northwestern Engineering’s Ken Forbus, Walter P. Murphy Professor of Computer Science and roadmap workshop chair. The event featured an impressive list of panelists that included:
- V.S. Subrahmanian, Walter P. Murphy Professor of Computer Science at the McCormick School of Engineering and faculty fellow at the Northwestern Buffett Institute for Global Affairs
- Eric Horvitz, chief scientific officer at Microsoft
- Anand Rao, the global AI lead and US innovation lead for the Emerging Technology Group at PwC US
- Bart Selman, professor of computer science at Cornell University
- Manuela Veloso, the head of AI research at J.P. Morgan and professor at Carnegie Mellon University
Subrahmanian has spent the past 15 years studying how AI can identify malicious actors, predict what they’ll do, and then use predictive models to anticipate or change the conditions so those bad actors are less likely to succeed.

Paraphrasing Sun Tzu, who said, ‘if you know the enemy and know yourself, you will win the next 100 battles,’ Subrahmanian said if you know yourself but not the enemy, you’re basically going to win some and lose some. And if you don’t know both yourself and the enemy, Subrahmanian added, you’re not going to win a whole lot.
That sentiment is applicable in our everyday lives and applying AI.
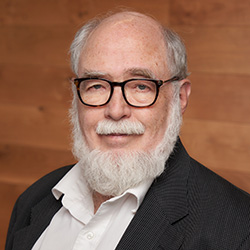
When people try to convince someone to take their position, they’re making an argument based on precedent and analysis of both the data involved and how the other person will respond go a given argument. It’s important to stress why you’re right, and what the consequences are if your predictions are right or wrong. Understanding that – and the subject’s cognitive state, biases, and preferences is key, and that requires linguistic, behavioral, and logical reasoning on top of predictive models.
“I love machine learning and all the stuff that it does, but it’s not the only part of the picture,” Subrahmanian said. “These explanations have to be rendered and couched in ways that require even more AI.”
That’s why it’s key for stakeholders to have faith in the AI they’re using.
More strategic, trustworthy AI systems
Rao said the business world sees strategy as uniquely human. Replicating the ability to plot a return on investment, figure out the right customer segments, and calculate how much to invest are challenges for AI. Most of AI’s use is around operational decisions, not helping humans in strategic decisions. Rao said building trustworthy, unbiased AI systems is imperative – and that trust must be earned from consumers.

“Addressing it not just as a technical issue, because I don’t think it’s totally a technical issue, addressing it as a social technical system I think is key,” Rao said. “I think we should be spending more time in that area to make sure we can earn and keep the trust of consumers.”
Exciting times
Horvitz said researchers have made significant strides in representation and inference methods since the AI field took shape in the late 1950s. There have been jumps in competency in natural language and perceptual tasks during the last decade, but more work remains.
There have only been small advances in the understanding of core aspects of intelligence, and little progress on the computational foundations of the multiple mysteries of the human intellect and what it can achieve.
“All of these incredible powers are still almost complete mysteries,” Horvitz said. “Despite our poor understanding, their incredible existence proves they define aspirations about the possibilities of better understanding their principles and the mechanisms underlying intelligence. It’s a very exciting time to be engaged with AI research.”
The right investment targets
Where the research is being targeted, though, could be tweaked.
Selman, one of the roadmap’s co-chairs, said he’s concerned that funding for core AI research is lagging behind investments into deep-learning and data-driven AI. The latter, Selman said, are almost too effective at what they do.
He referenced how in 1997 IBM’s Deep Blue computer defeated world champion Garry Kasparov in chess using tactics not utilized by humans. Since then, AI systems have been taught to play chess in a more human-like way. Teaching AI systems how to do tasks interactively, rather than by trial and error, could lead to AI systems that go beyond the limitations of data-driven AI. Selman observed that companies may well invest in data-driven AI for the near term. However, countries that want to advance AI should, Selman argued, take a longer view and invest in cognition-based AI.
“We have to convince the broader public and policymakers that this is still a real issue,” Selman said.
AI interactions
Veloso has worked to connect reasoning with perception and data and planning for decision-making and executing plans in the real world. This loop, and trying to close it, has fascinated Veloso during her career. Veloso said she cares about humans but doesn’t care about creating information just for humans to see; she wants to digitize information so AI systems can make plans and execute them accordingly.
AI, Veloso said, is not about a single mind, but rather dependent on how algorithms from multiple entities work together, and becomes more complicated when AI systems from one company interact with another. She also stressed the importance of AI being a system that continuously learns from past experiences.
“At any moment, AI systems have their own limitations, but can they include feedback? Can they actually become better if you interact with them?” she asked. “That’s what we should have in our hearts.”